Joseph Nelson, co-founder and CEO of Roboflow, discusses how the company is making computer vision accessible to millions of developers and industries, from manufacturing to healthcare and more.
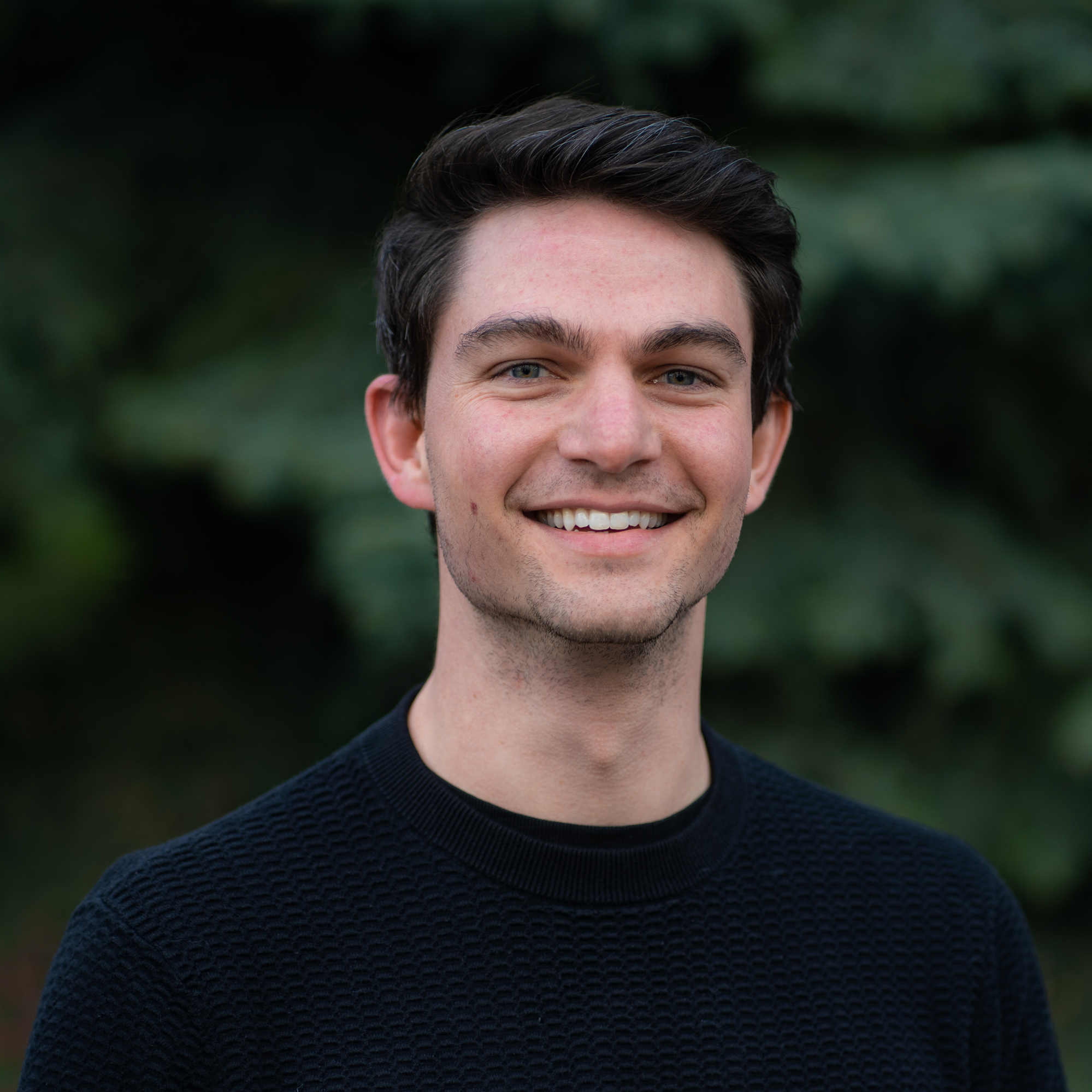
Joseph Nelson, co-founder and CEO of Roboflow, discusses how the company is making computer vision accessible to millions of developers and industries, from manufacturing to healthcare and more.